AI explainability and the future of regulation
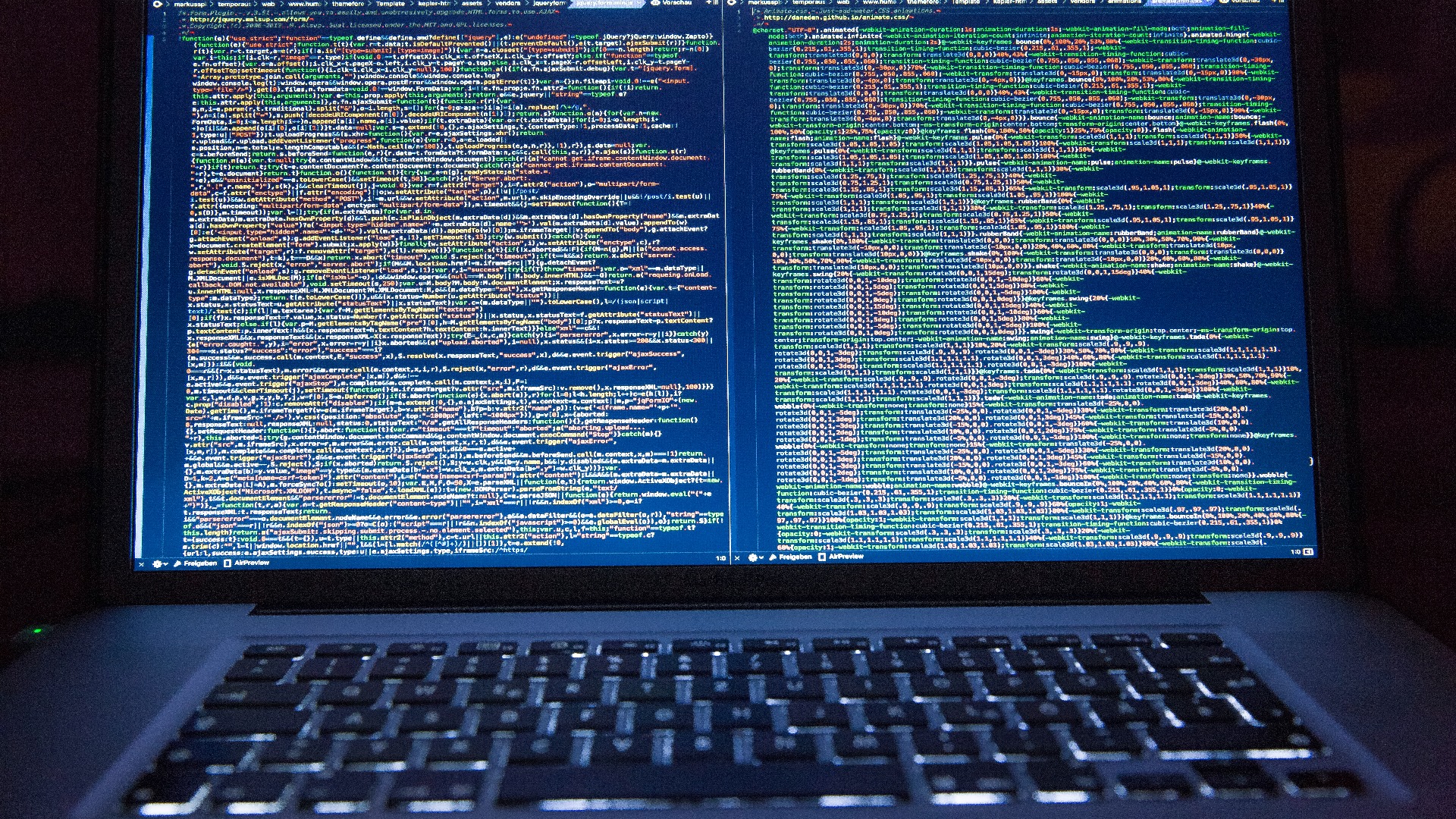
- Central to all AI regulation will be explainability of AI systems.
- The UK government defines AI explainability as the extent to which it is possible for relevant parties to access, interpret, and understand the decision-making process of an AI system.
- Explainability is important to ensure that the outcomes of AI systems are fair and unbiased, and they don’t breach existing regulations, e.g., Equality Act, 2010.
- At Fuse we obey four key principles to ensure AI explainability.
What is explainability in AI?
To ensure that uses of artificial intelligence (AI) systems are beneficial and non-harmful, governing bodies around the world have started to set requirements. Among these requirements is ‘explainability’ - others include transparency, fairness, and security.
Explainability has been defined slightly differently from different governing bodies:
- The UK government says explainability “refers to the extent to which it is possible for relevant parties to access, interpret, and understand the decision-making process of an AI system.”
- The OECD claims “explainability means enabling people affected by the outcome of an AI system to understand how it was arrived at.”
- The European Commission says that explainability involves the decisions and predictions being understood by humans, and not violating its core set of assumptions and principles.
There are slight differences in these definitions. Generally speaking the UK government’s definition is the loosest. This is to be expected, as the main aim of the government’s white paper was to encourage innovation in the AI space, not limit uses.
Yet, they all share a common theme - human understanding and intervention should be possible at all stages of an AI system. In particular, those who are stakeholders in the AI system’s decision - the suppliers, distributors, and the person directly affected.
Why should I care about AI explainability?
The pragmatic answer as to why you should care is that it will be part of the law soon. It is likely that new regulations will be made to supplement existing laws that might cover AI use, such as the Equality Act, 2010. If you are using AI systems, making them explainable will help preempt these changes.
There are non-pragmatic reasons, too. To ensure your systems aren’t making biassed decisions, you need to be able to demonstrate why your algorithms are fair. Furthermore, explainability will help build public trust in decisions made by AI systems.
Explainability is also a means to an end for some of the other AI principles suggested in regulation. It is difficult to know if your system is suitably secure, for example, unless you are able to break down the data sources and algorithms involved in a decision.
Potential future directions for regulation
Regulation is nascent, and hence not very prescriptive. It will, though, become necessary to ensure your AI systems are explainable.
At Fuse, we have created four key principles to ensure our AI is explainable at all stages of the value chain. These are:
- Human intervention
- Comprehensible explanations
- Data transparency
- Bias limitation
These principles also serve as a prediction for what regulatory requirements might look like. Let’s run through each:
Human intervention involves the ability to monitor automated decisions and intervene where necessary.
Comprehensible explanations entails understandability for the recipient of the outcome. This means being able to explain to them in plain English why a specific decision was made - with reference to the algorithm and principles underpinning them.
Data transparency is about clearly labelled input data - both for training the model, and for specific outcomes - in order to trace potential bias.
Bias limitation requires a set of core assumptions decided prior to model creation which cannot be breached, ensuring fair outcomes.
We ensure all four of these principles are met by having ex-ante explainable models - i.e., models which are explainable prior to it being fit on the training data. The relationships between variables are already decided with an expert in the loop. This is particularly important for sensitive attributes like age or gender, which are accounted for correctly. Hence if they are built to be unbiased at this stage, then applied to data no accidental biases should occur. If biases were to appear, or we want to enquire after training, each variable can be controlled for, in order to see how the outcome varies.
If you want to preempt AI regulation, you should look to follow a set of principles to ensure your AI systems are explainable. If you want to find out more about our models, demo them today by booking a calendar slot.